CyberAware: Secure AI Usage
- teena420
- Mar 25
- 6 min read
What is AI security?
Short for artificial intelligence (AI) security, AI security is the process of using AI to enhance an organization's security posture. With AI systems, organizations can automate threat detection, prevention and remediation to better combat cyberattacks and data breaches.
Organizations can incorporate AI into cybersecurity practices in many ways. The most common AI security tools use machine learning and deep learning to analyze vast amounts of data, including traffic trends, app usage, browsing habits and other network activity data.
This analysis allows AI to discover patterns and establish a security baseline. Any activity outside that baseline is immediately flagged as an anomaly and potential cyberthreat, allowing for swift remediation.
What does secure AI usage mean?
In terms of AI security, Secure AI usage refers to the set of practices, strategies, and technologies that ensure AI systems are used safely, ethically, and reliably, while mitigating the risks associated with their deployment and use. It involves protecting AI systems from threats that could compromise their integrity, safety, and the security of the data they process. Essentially, Secure AI usage focuses on preventing malicious attacks, unauthorized access, data breaches, and other vulnerabilities that could exploit AI systems.
NIST Cybersecurity Framework
Cybersecurity puts policies, procedures and technical mechanisms in place to protect, detect, correct and defend against damage, unauthorized use or modification, or exploitation of information and communication systems and the information they contain. The rapid pace of technological change and innovation, along with the rapidly evolving nature of cyber threats, further complicates the situation. In response to this unprecedented challenge, AI-based cybersecurity tools have emerged to help security teams efficiently mitigate risks and improve security.
Given the heterogeneity of AI and cybersecurity, a uniformly accepted and consolidated taxonomy is needed to examine the literature on applying AI for cybersecurity. This structured taxonomy will help researchers and practitioners come to a common understanding of the technical procedures and services that need to be improved using AI for the implementation of effective cybersecurity.
For this purpose, a well-known cybersecurity framework proposed by NIST was used to understand the solution categories needed to protect, detect, react and defend against cyberattacks. The NIST cybersecurity framework's core describes the practices to improve the cybersecurity of any organization. The framework's core has four elements: Functions, Categories, Subcategories and Informative references.
The first two levels of the NIST framework, which consist of 5 cybersecurity functions and 23 solution categories, were used to classify the identified AI use cases.
The functions provide a comprehensive view of the lifecycle for managing cybersecurity over time. The solution categories listed under each function offer a good starting point to identify the AI use cases to improve the cybersecurity. The main purpose of selecting these two levels is to provide a clear and intuitive categorization to classify the existing AI for cybersecurity literature into the appropriate solution category. The proposed taxonomy introduces a third level consistent with the first two levels by specifying AI-based use cases corresponding to each level of the cybersecurity framework
NIST Cyber Security Framework Diagram

Why AI security is important?
Today's cyberthreat landscape is complex. The shift to cloud and hybrid cloud environments has led to data sprawl and expanded attack surfaces while threat actors continue to find new ways to exploit vulnerabilities. At the same time, cybersecurity professionals remain in short supply, with over 700,000 job openings in the US alone.
AI security can offer a solution. By automating threat detection and response, AI makes it easier to prevent attacks and catch threat actors in real time. AI tools can help with everything from preventing malware attacks by identifying and isolating malicious software to detecting brute force attacks by recognizing and blocking repeated login attempts.
With AI security, organizations can continuously monitor their security operations and use machine learning algorithms to adapt to evolving cyberthreats.
Types of AI-based Cybersecurity Tools in maintaining Data Privacy & Security

Challenges in Implementing AI security
Data Privacy and Security Concerns: AI requires large datasets, which may involve sensitive information, raising privacy and security risks.
Adversarial Attacks on AI Models: AI models can be manipulated by adversarial attacks, bypassing detection and compromising security.
Data Quality and Bias: Poor-quality or biased data can lead to inaccurate predictions and flawed decision-making in AI systems.
Integration with Existing Security Infrastructure: Integrating AI with legacy systems can be complex, requiring adjustments and compatibility checks.
Cost and Resource Requirements: Implementing AI security tools can be costly and resource-intensive, especially for small to medium-sized organizations.
Lack of Skilled Workforce: There is a shortage of skilled professionals with expertise in both AI and cybersecurity.
Ethical and Regulatory Challenges: AI systems may raise privacy, ethical, and legal concerns, especially in areas like surveillance and data use.
Complexity and Overfitting of AI Models: AI models may overfit on training data, resulting in poor performance in real-world scenarios.
Lack of Transparency (Black Box Nature): Many AI models are opaque, making it difficult to interpret their decision-making processes.
Continuous Monitoring and Adaptation: AI models need regular updates and monitoring to adapt to the continuously evolving threat landscape.
Future Aspects of AI Security
The future aspects of AI security are exciting and transformative, focusing on advancing protection mechanisms, enhancing automation, and addressing emerging challenges. Here are some examples of key future aspects:
1. Predictive Threat Intelligence
AI will evolve into predictive cybersecurity, moving beyond reactive defense to anticipate threats before they occur. By analyzing vast amounts of historical and real-time data, AI will predict attack patterns and proactively mitigate risks.
2. Improved Behavioral Analytics
AI will refine its ability to detect anomalies in user behavior by analyzing patterns such as logins, device activity, and data access. This will enhance the Zero Trust model, where access and trust are constantly verified and adjusted based on behavior.
3. AI in Threat Simulation and Red Teaming
AI will play a major role in red teaming (simulated cyberattacks) by mimicking potential adversaries and testing security defenses. These AI-driven simulations will be more dynamic and realistic, providing organizations with advanced insights into vulnerabilities.
The future of AI security is shaped by the need for smarter, faster, and more adaptive defense mechanisms. With advancements like predictive intelligence, autonomous defenses, and AI-enhanced threat simulations, AI will become an even more essential part of cybersecurity. However, as AI systems evolve, they must be ethically designed and aligned with privacy standards to ensure a balanced and secure digital future.
AI security best practices
To balance AI’s security risks and benefits, many organizations craft explicit AI security strategies that outline how stakeholders should develop, implement and manage AI systems.
While these strategies necessarily vary from company to company, some of the commonly used best practices include:
1. Establishing formal data governance procedures
Implementing data governance and risk management strategies is essential to safeguard sensitive information utilized in AI processes, all while preserving the effectiveness of AI.
By ensuring the use of relevant, high-quality training datasets and consistently updating AI models with fresh data, organizations can better ensure that their models remain adaptable to emerging threats.
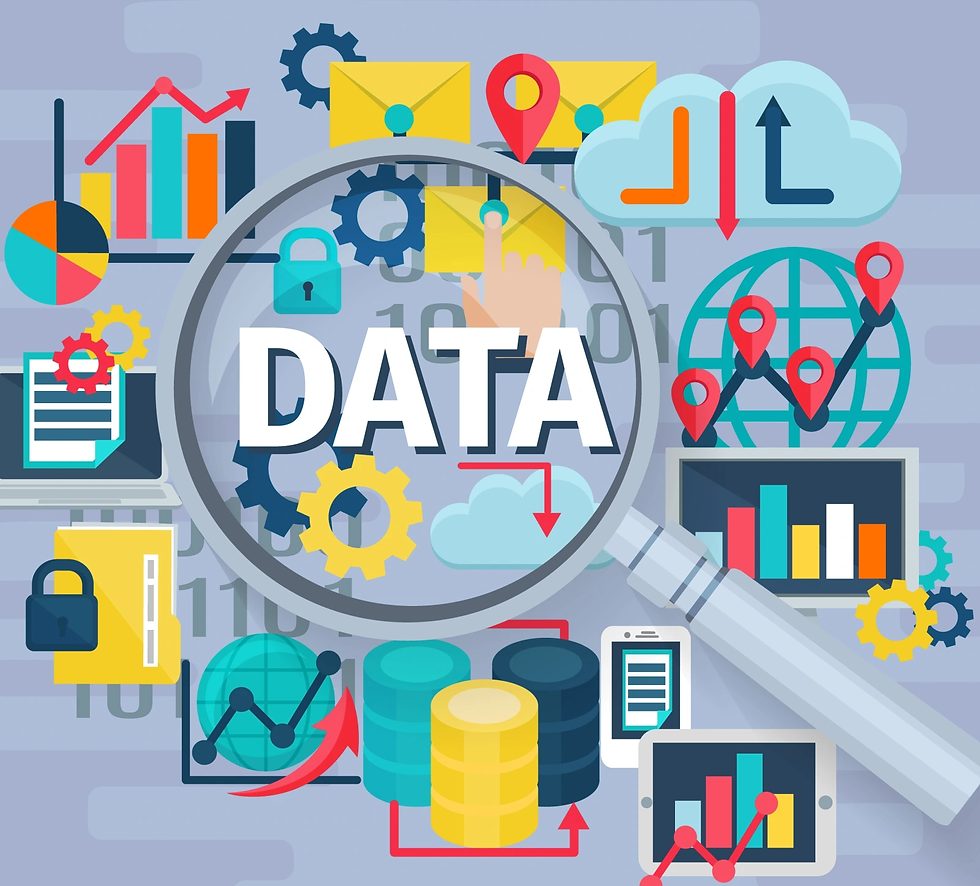
2. Integrating AI with current security systems
Connecting AI tools with existing cybersecurity frameworks, such as threat intelligence sources and SIEM (Security Information and Event Management) systems, can enhance their effectiveness and reduce disruptions or downtime that may occur when introducing new security technologies.
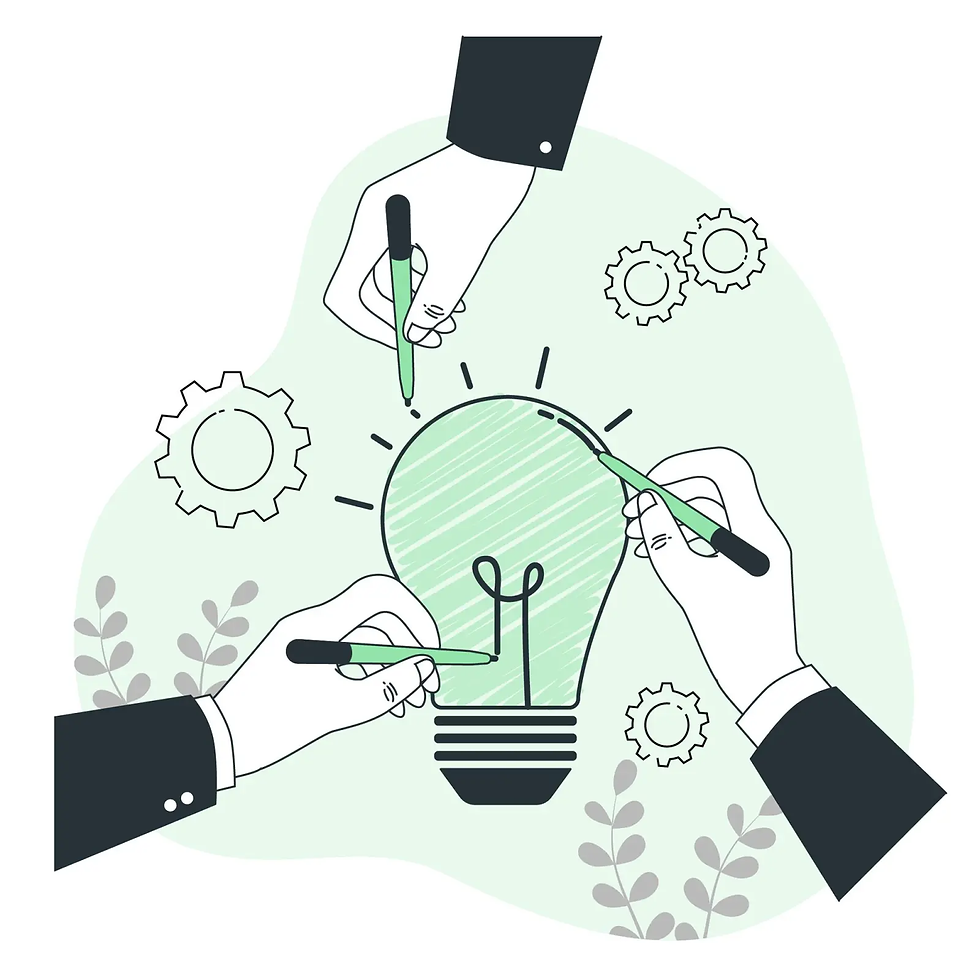
3. Focusing on ethics and transparency
Ensuring transparency in AI systems by documenting algorithms and data sources and keeping open lines of communication with stakeholders about AI usage can help uncover and address potential biases and issues of fairness.
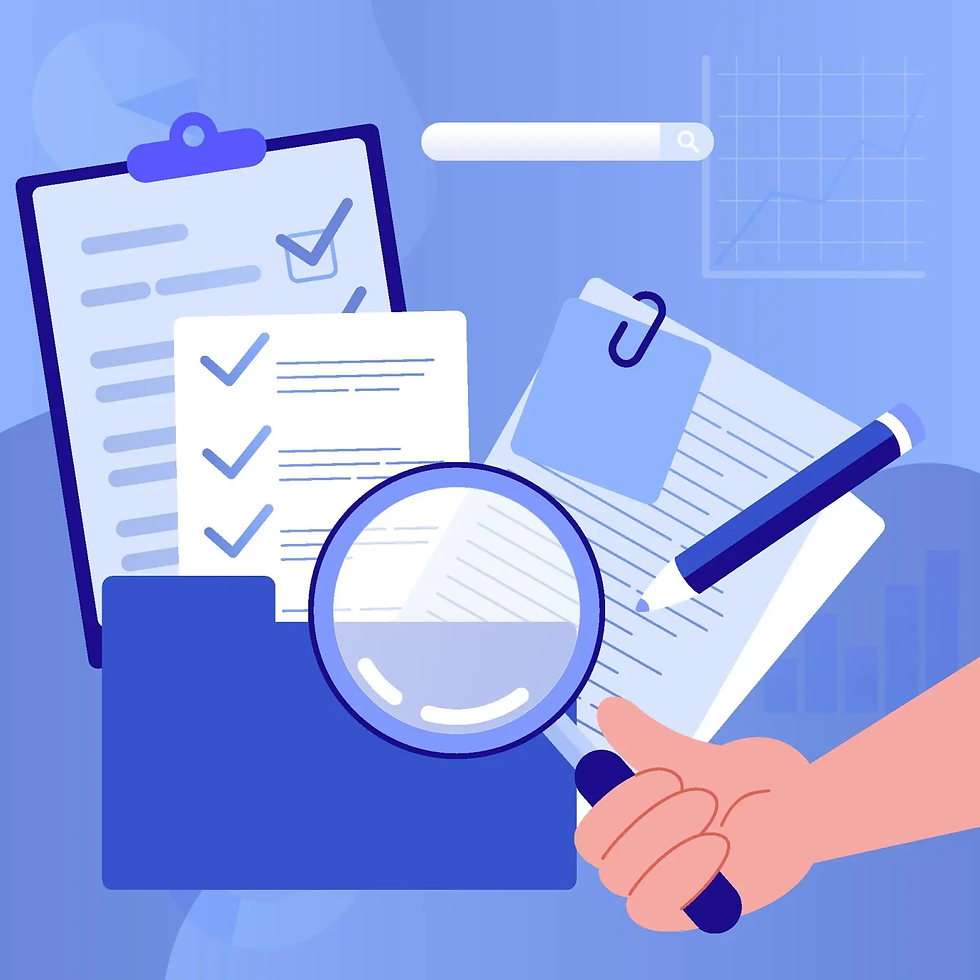
4. Implementing security measures for AI systems
Though AI technologies can strengthen security, they also require their own protective measures.
Using encryption, access controls, and threat detection tools can help organizations safeguard their AI systems and the sensitive data they process.
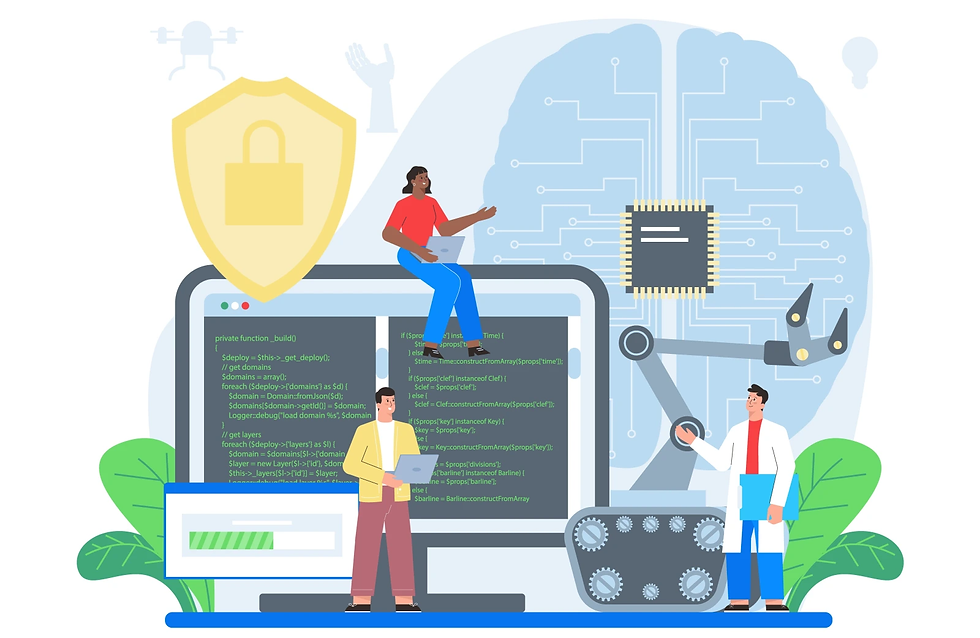
5. Ongoing monitoring and assessment
Regularly assessing AI systems for performance, compliance, and accuracy is crucial for meeting regulatory standards and refining AI models over time.
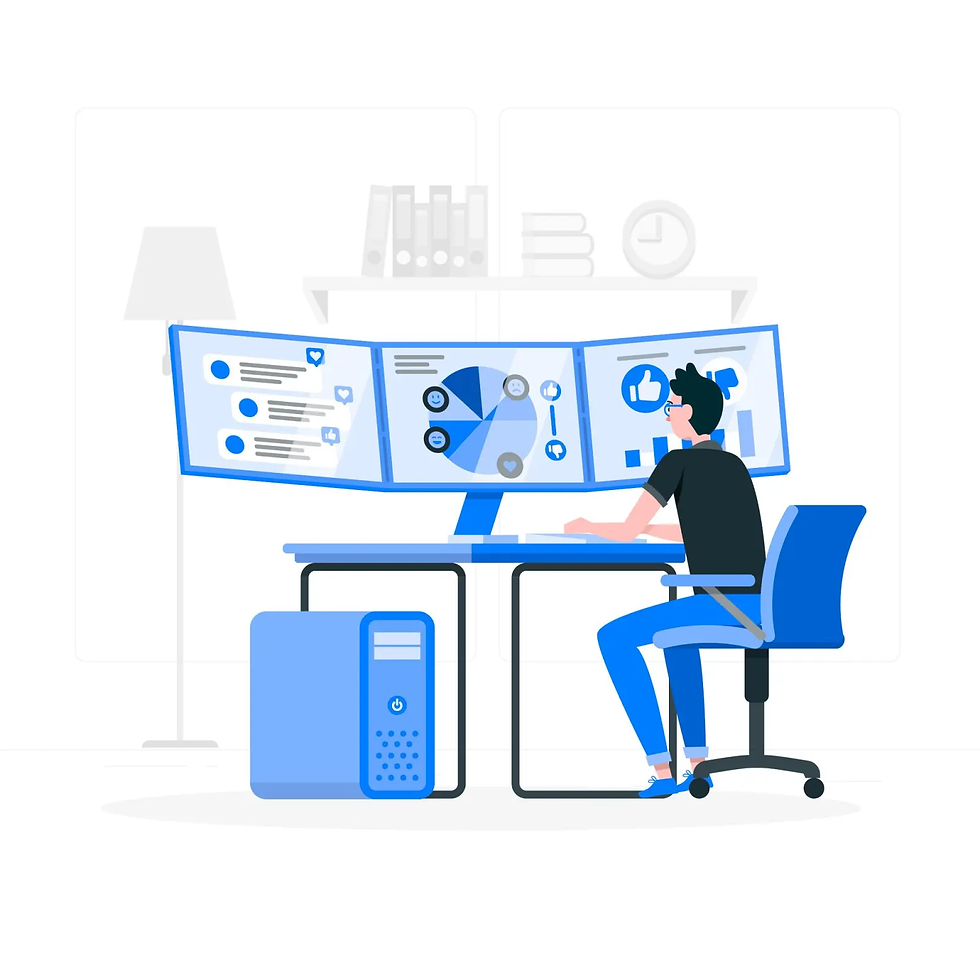
References:
What is AI security? - What is AI Security? - IBM
The Role of Machine Learning in Cybersecurity - Role of ML in Cybersecurity
The state of AI: How organizations are rewiring to capture value -The State of AI - mckinsey
AI in Data Privacy & Security - AI in Data Privacy & Security
AI-enabled cybersecurity and Legal frameworks adaption - AI-enabled cybersecurity and Legal frameworks
Artificial intelligence for cybersecurity: Literature review and future research directions -AI for Cyber Security