We have, in our previous blogs, taken the time to go down deep into the different benefits that AI brings, the pain points that CEOs have to solve by optimizing operations to accessing value in unstructured data. With AI’s ability to improve efficiency, decision-making, and productivity at large, one would think that huge adoption across industries will happen and is happening; that’s not the case, though.
According to PwC’s Emerging Technology Survey, 73% of US companies are using AI in at least some areas of their operations. In just the first year following ChatGPT’s release, more than half (54%) of the companies surveyed had incorporated parts of their business with GenAI.
Everybody knows that AI brings a lot of obvious benefits. Yet, most of the CEOs remain reluctant to fully embracing AI within their companies, especially generative AI tools. Why? If the potential for success seems so obvious, why are these tools not more widely adopted yet? What are the barriers that stand in the way of it’s wide-scale adoption and what, consequently, can be done to increase confidence in AI-driven solutions?
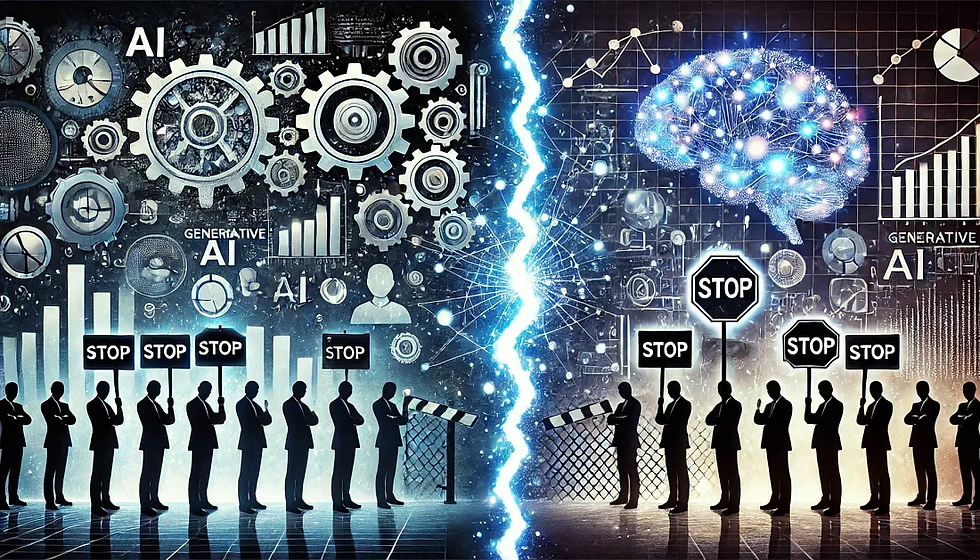
Let’s understand the major concerns for CEO’s today and propose solutions for each of them to endorse GenAI in their businesses.
1. Unclear Return on Investment (ROI)
Challenge: Most CEOs do not want to invest in GenAI mainly because of uncertainty of ROI. Although the technology has tremendous promise, most direct ROI for most industries cannot be clearly articulated, especially in industries that are unaware of how AI applies to them. CEOs may think the investment will not bring any kind of payback, especially in the short-term period.
Solution: Companies should start with controlled small pilots to implement GenAI, which clearly state objectives and KPIs. The controlled pilot environment provides an opportunity for companies to test its potential in given areas, assess the outcome, and adjust their strategy from measurable result perspectives. This can reduce some of the risks and offer faster return on investment in case the company decides to collaborate with AI consultants or pre-built AI solutions specifically designed for the industry.
2. High Implementation Costs
Challenge: Cost of adoption or the cost of implementing GenAI is high. It is not just the cost of software and infrastructure investments but also the hiring and training of talent, support, and the cost of integrating AI into the existing system. That’s terrifying to CEOs in uncertain economic environments.
Solution: Scalable cloud-based GenAI solutions pay-per-use models reduce upfront costs. Subscription-based models are flexible and help cut down capital expenditure. Additionally, businesses should look to co-investment with technology partners in GenAI initiatives or explore AI-as-a-Service models wherein most internal resources often required for deployment are either minimal or less.
3. AI projects stalling at PoC stage
Challenge: The most common issue presented is when AI project ideas get stuck at the Proof of Concept (PoC) stage without being brought up to full production, an experience many CEOs have had. Often, the reasons appear to be multifaceted, so the approach to the solution must be strategic.
According to Gartner Inc. at least 30 percent of generative AI projects that have proven their concept will fail by the end of 2025 due to poor data quality, inadequate risk controls, ballooning costs or vague business value.
Solution: To prevent AI projects stalling at PoC stage, CEOs must have strong business value in starting points, aligned projects for measurable outcomes, and the quality of data through strong governance. Risks should be addressed early with good compliance and ethical frameworks set up, cost control through scalable iterative deployments and avoid blowout; reduction of resistance by having the right change management, reskilling, and clear communications with all stakeholders involved. Momentum and thrust to full production are gained, hence maximizing value, reducing failure risks, by continuous leadership support and cross-functional involvement.
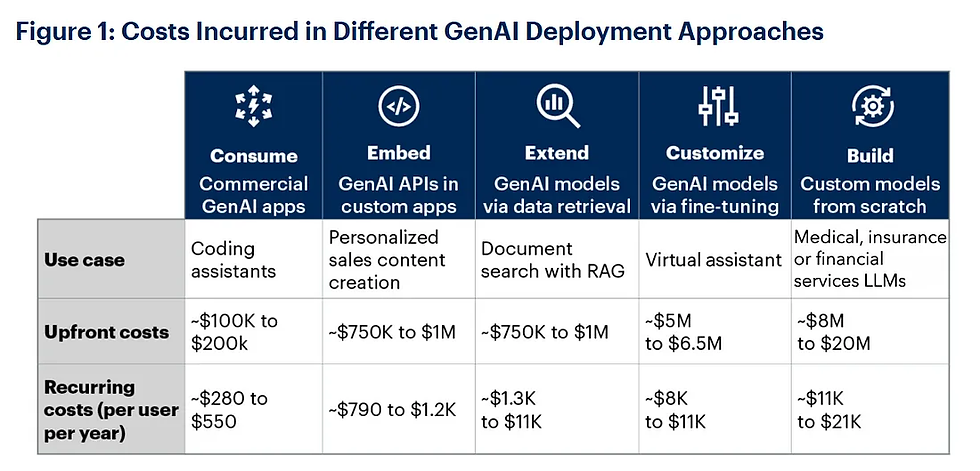
4. Lack of Expertise
Challenge: Most organizations do not have in-house capabilities that ensure the proper deployment and operations of GenAI solutions. This fact has made CEOs anxious about their chances of succeeding in AI projects because without the proper talent, they are exposed to a higher probability of failure.
Solution: The gap between this potential source of innovation and the actual utilization of AI consulting firms or hiring specialized AI contractors, or even forming collaborations with universities and research institutions, should be closed by companies. Upskilling and training programmes for existing staff, especially in AI project management, would further facilitate internal capabilities for businesses. No technical expertise would be required to utilize gen AI platforms through user-friendly, no-code or low-code GenAI, empowering non-experts.
5. Data Privacy and Security Concerns
Challenge: Sensitive information used by GenAI could be a real threat to privacy and security. The generative AI models require huge amounts of data, thus companies risk data breaches, non-compliance with regulation, and misuse of personal information.
Solution: A strong data governance framework can be set for data protection and security. Technologies that might help- encryption, anonymization, and access controls. Another alternative would be fantastic when AI solutions are deployed on premises or hybrid cloud models where there is a serious concern taken over data. One has to give importance to compliance to laws such as GDPR or HIPAA, and regular audits with third-party security reviews make the CEOs feel more comfortable about adoption.
6. Workforce Displacement Issues
Challenge: CEOs fear that the application of GenAI will displace peoples’ jobs and potentially workers on large workforces. The fear of displacement of workers leaves cases of resistance among employees to the new change, making PR a nightmare. CEOs have yet another concern related to broader social and economic policy implications since the tasks to be automated would have traditionally been performed by people.
Solution: In reality, the adoption frames of GenAI should be designed in a way that it assists companies avoid replacing human labor, instead actually augmenting it. A well-defined reskilling and retraining program provided by the business will enable employees to shift into other roles that concentrate on creative, strategic, or high-value work that complements AI. Alarmism and fears over the displacement of jobs can only be assuaged through a clear communication strategy on how AI would help increase efficiency and encourage people to focus on more fulfilling work.
7. Regulatory and Ethical Uncertainty
Challenge: The regulatory landscape of AI is at its infancy, with multiple CEOs fearing some future government regulations or judicial consequences. Second, ethical concerns in AI decisions, bias in AI outputs, or unintended consequences make the implementation of GenAI a significant risk for the CEO, especially in health care, finance, or law industries.
Solution: Companies should establish AI ethics committees or hire compliance experts knowledgeable about both present and future regulations requiring them to be transparent, fair, and compliant with their use of AI. Forward thinking to collaborate with regulatory policymakers, industry organizations, and AI governance efforts could also place companies ahead of regulatory adjustments. In addition, there should be an effort to build more transparent, explainable AI models, which would be easier to audit and verify as fair and accountable.
8. Quality and Accuracy Issues
Challenge: While generative AI models can create text that is really creative, it’s prone to fact errors, biased outcomes, or, more simply, lousy output quality. CEOs would worry about reputation risks and operational inefficiencies inherent in a system so imperfect.
Solution: Quality issues can be dealt with thorough enforcing of human-in-the-loop systems where each output produced by AI is reviewed and validated by the presence of humans before the outputs are deployed or shared publicly. Businesses can also train AI models on domain-specific data to make it more precise and relevant. AI system and performance metrics audits at regular time intervals ensure that the models continue to improve and stay quality bound.
9. Cultural Resistance
Challenge: The introduction of GenAI may also face culture resistance from the organizations. The new workflows may not be so pleasant for the employees who are not used to the work of AI, fearing that AI could be the end of their employment or uproot their environment of work. In such cases, most CEOs feel reluctant to force it down on their staff first.
Solution: The company should work on the AI adoption strategy, which should come through strong inside communication and manage the change within with initiatives towards employee engagement. The culture of acceptance has to be established in a workshop, training session, and open forums where employees learn about AI and its concerns. Early success with a demonstration of how AI helps eliminate mundane tasks can demonstrate the effect of AI in a positive way on the employees.
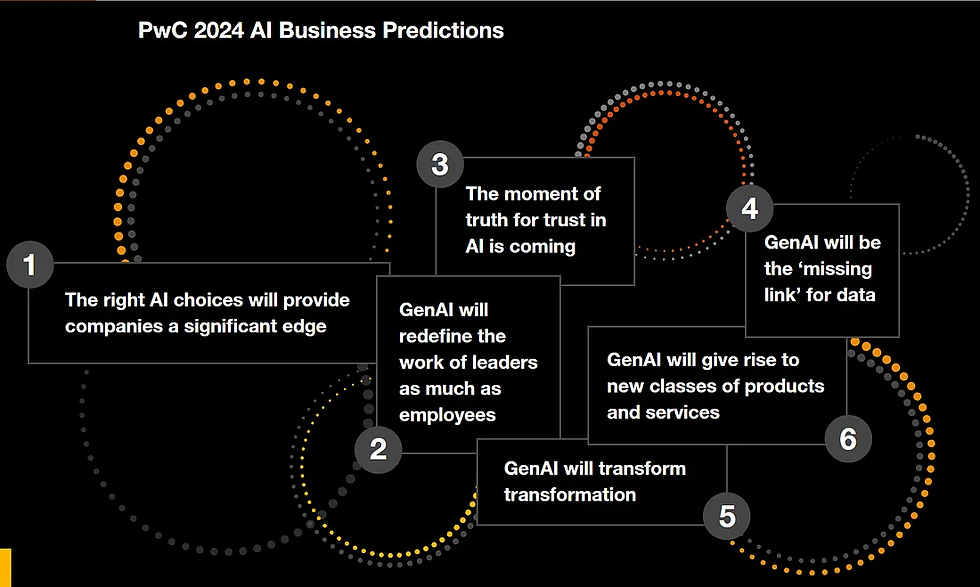
Conclusion
With these worries being tackled strategically, CEOs can start feeling more confident about embracing GenAI, and then begin to introduce it in their business, thus leading to improvements in innovation and operational efficiency.
Giants like PwC in their recent article mentioned on the future of AI- “We’re already seeing cloud-based enterprise applications incorporate more GenAI capabilities, but this is just the start. Soon, enterprise applications will have GenAI not as an add-on but as the core.”
AI contains transformative potential, through which it can enhance efficiency, improve decisions, and increase productivity. Still, many are slow in the adoption of GenAI. There is reluctance by the CEOs, largely based on ROI and implementation costs, expertise, data privacy, and the uncertainty of current regulations.
However, we’ve demonstrated how each problem can be overcome with strategic solutions. Organizations can counter all these concerns from small pilots to using scalable, cloud-based AI models. Upskilling, governance frameworks, and reinforcing collaborative coexistence between AI and human labor also smoothen the integration process.
In conclusion, if companies create very clear strategies and move proactively, they may embrace AI without further ado, unlock new and higher levels of innovation, and achieve greater operational efficiency. Now is the time for action, lest laggards miss massive opportunities through AI.